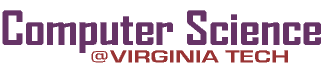
Autoregressive Models of Background Errors for Chemical Data Assimilation
2006) Autoregressive Models of Background Errors for Chemical Data Assimilation. Technical Report TR-06-22, Computer Science, Virginia Tech. (
Full text available as: |
Abstract
The task of providing an optimal analysis of the state of the atmosphere requires the development of dynamic data-driven systems that efficiently integrate the observational data and the models. Data assimilation (DA) is the process of adjusting the states or parameters of a model in such a way that its outcome (prediction) is close, in some distance metric, to observed (real) states. It is widely accepted that a key ingredient of successful data assimilation is a realistic estimation of the background error distribution. This paper introduces a new method for estimating the background errors which are modeled using autoregressive processes. The proposed approach is computationally inexpensive and captures the error correlations along the flow lines.
Item Type: | Departmental Technical Report |
---|---|
Keywords: | background covariance, data assimilation, autoregressive models |
Subjects: | Computer Science > Numerical Analysis |
ID Code: | 926 |
Deposited By: | Constantinescu, Mr. Emil M. |
Deposited On: | 12 October 2006 |