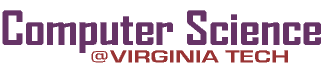
Simultaneous Optimal Uncertainty Apportionment and Robust Design Optimization of Systems Governed by Ordinary Differential Equations
2011) Simultaneous Optimal Uncertainty Apportionment and Robust Design Optimization of Systems Governed by Ordinary Differential Equations . Technical Report TR-11-15, Computer Science, Virginia Tech. (
Full text available as: |
Abstract
The inclusion of uncertainty in design is of paramount practical importance because all real-life systems are affected by it. Designs that ignore uncertainty often lead to poor robustness, suboptimal performance, and higher build costs. Treatment of small geometric uncertainty in the context of manufacturing tolerances is a well studied topic. Traditional sequential design methodologies have recently been replaced by concurrent optimal design methodologies where optimal system parameters are simultaneously determined along with optimally allocated tolerances; this allows to reduce manufacturing costs while increasing performance. However, the state of the art approaches remain limited in that they can only treat geometric related uncertainties restricted to be small in magnitude. This work proposes a novel framework to perform robust design optimization concurrently with optimal uncertainty apportionment for dynamical systems governed by ordinary differential equations. The proposed framework considerably expands the capabilities of contemporary methods by enabling the treatment of both geometric and non-geometric uncertainties in a unified manner. Additionally, uncertainties are allowed to be large in magnitude and the governing constitutive relations may be highly nonlinear. In the proposed framework, uncertainties are modeled using Generalized Polynomial Chaos and are solved quantitatively using a least-square collocation method. The computational efficiency of this approach allows statistical moments of the uncertain system to be explicitly included in the optimization-based design process. The framework formulates design problems as constrained multi-objective optimization problems, thus enabling the characterization of a Pareto optimal trade-off curve that is off-set from the traditional deterministic optimal trade-off curve. The Pareto off-set is shown to be a result of the additional statistical moment information formulated in the objective and constraint relations that account for the system uncertainties. Therefore, the Pareto trade-off curve from the new framework characterizes the entire family of systems within the probability space; consequently, designers are able to produce robust and optimally performing systems at an optimal manufacturing cost. A kinematic tolerance analysis case-study is presented first to illustrate how the proposed methodology can be applied to treat geometric tolerances. A nonlinear vehicle suspension design problem, subject to parametric uncertainty, illustrates the capability of the new framework to produce an optimal design at an optimal manufacturing cost, accounting for the entire family of systems within the associated probability space. This case-study highlights the general nature of the new framework which is capable of optimally allocating uncertainties of multiple types and with large magnitudes in a single calculation.
Item Type: | Departmental Technical Report |
---|---|
Keywords: | Uncertainty Apportionment, Tolerance Allocation, Robust Design Optimization, Dynamic Optimization, Nonlinear Programming, Multi-Objective Optimization, Multibody Dynamics, Uncertainty Quantification, Generalized Polynomial Chaos |
Subjects: | Computer Science > Mathematical Software |
ID Code: | 1163 |
Deposited By: | Hays, Joe |
Deposited On: | 31 January 2013 |