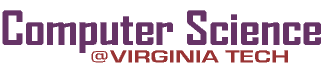
Space-time adaptive solution of inverse problems with the discrete adjoint method
2010) Space-time adaptive solution of inverse problems with the discrete adjoint method. Technical Report TR-10-14, Computer Science, Virginia Tech. (
This is the latest version of this eprint.
Full text available as: |
Abstract
Adaptivity in both space and time has become the norm for solving problems modeled by partial differential equations. The size of the discretized problem makes uniformly refined grids computationally prohibitive. Adaptive refinement of meshes and time steps allows to capture the phenomena of interest while keeping the cost of a simulation tractable on the current hardware. Many fields in science and engineering require the solution of inverse problems where parameters for a given model are estimated based on available measurement information. In contrast to forward (regular) simulations, inverse problems have not extensively benefited from the adaptive solver technology. Previous research in inverse problems has focused mainly on the continuous approach to calculate sensitivities, and has typically employed fixed time and space meshes in the solution process. Inverse problem solvers that make exclusive use of uniform or static meshes avoid complications such as the differentiation of mesh motion equations, or inconsistencies in the sensitivity equations between subdomains with different refinement levels. However, this comes at the cost of low computational efficiency. More efficient computations are possible through judicious use of adaptive mesh refinement, adaptive time steps, and the discrete adjoint method. This paper develops a framework for the construction and analysis of discrete adjoint sensitivities in the context of time dependent, adaptive grid, adaptive step models. Discrete adjoints are attractive in practice since they can be generated with low effort using automatic differentiation. However, this approach brings several important challenges. The adjoint of the forward numerical scheme may be inconsistent with the continuous adjoint equations. A reduction in accuracy of the discrete adjoint sensitivities may appear due to the intergrid transfer operators. Moreover, the optimization algorithm may need to accommodate state and gradient vectors whose dimensions change between iterations. This work shows that several of these potential issues can be avoided for the discontinuous Galerkin (DG) method. The adjoint model development is considerably simplified by decoupling the adaptive mesh refinement mechanism from the forward model solver, and by selectively applying automatic differentiation on individual algorithms. In forward models discontinuous Galerkin discretizations can efficiently handle high orders of accuracy, $h/p$-refinement, and parallel computation. The analysis reveals that this approach, paired with Runge Kutta time stepping, is well suited for the adaptive solutions of inverse problems. The usefulness of discrete discontinuous Galerkin adjoints is illustrated on a two-dimensional adaptive data assimilation problem.
Item Type: | Departmental Technical Report |
---|---|
Keywords: | automatic differentiation, discrete adjoint method, adaptive mesh refinement, Runge-Kutta methods, discontinuous Galerkin methods, 4D-Var |
Subjects: | Computer Science > Numerical Analysis |
ID Code: | 1134 |
Deposited By: | Alexe, Mihai |
Deposited On: | 03 December 2010 |
Available Versions of this Item
- Space-time adaptive solution of inverse problems with the discrete adjoint method (deposited 03 December 2010) [Currently Displayed]